Accelerating AI/ML Value: Transforming Investment to Impact
Explore how to derive business impact, competitive advantage, and enhanced decision-making from AI/ML, and learn how to tackle the common challenges in achieving rapid time-to-value.
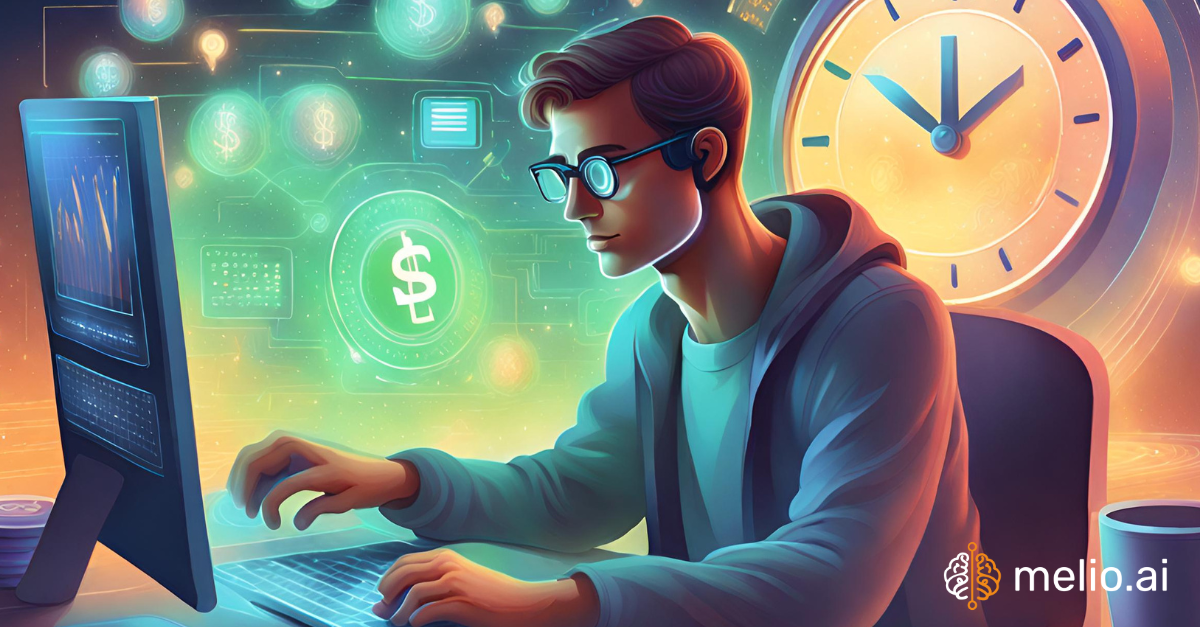
Photo by GPT4-vision.
In today’s business landscape, the race to leverage AI and ML for competitive advantage is more intense than ever. But here’s the million-dollar question: how do businesses translate AI/ML investments into tangible value swiftly and effectively?
But… what is Value?
Many companies face financial limitations that prevent them from undertaking costly and uncertain data science projects. Consequently, shortening the time to achieve value is a critical goal for data science leaders.
However, the concept of “value” needs clarification π.
Typically, “value” refers to whether there is a willingness to pay for a particular AI model, data pipeline, or set of features. In the context of AI/ML, reducing the time to value means quickly deriving benefits from data and analytics.
AI can deliver value in three main forms:
1οΈβ£ Business Impact (typically measured in financial terms):
To generate a positive business impact, companies should target manual and repetitive tasks that can be resolved by humans in less than five seconds. These tasks are ideal for AI automation, leading to cost savings and efficiency improvements.
π° Example: A bank implementing an AI platform that uses NLP to review and interpret commercial loan agreements, saving 360,000 hours of lawyer costs annually.
2οΈβ£ Competitive Advantage (e.g., market share, customer retention):
To enhance competitive advantage, companies can implement AI to differentiate their products or services, improve customer experience, and innovate faster than rivals, thereby gaining market share and enhancing customer loyalty.
π Example: An online learning platform employing AI-driven learning assistants to help students study more effectively. The Q&A style and automated assessments enhanced student engagement and boosted retention.
3οΈβ£ Enhanced Decision Making (measured by time saved):
To boost decision-making processes, companies can leverage AI to provide faster, data-driven insights, reducing the time spent on analysis and enabling quicker, more informed decisions.
β° Example: A hospital group analyses patient care data and prioritises nursing tasks within the hospital to reduce hospital-acquired infections.
Achieving value more rapidly leads to improved decision-making, enhanced efficiency, and maintaining a competitive edge in the marketplace. Therefore, the ability to swiftly identify use cases and extract value from data science initiatives is essential for companies to thrive in today’s competitive environment.
However, the journey isn’t always straightforward. Various challenges related to business strategies, processes, technology, and data can impede AI/ML projects. To truly accelerate AI adoption successfully, it’s crucial to recognize these potential obstacles early on and develop strategic approaches to overcome them.
Challenge 1: Business
Addressing business challenges is essential and should be the first step before starting any AI initiatives. After all, without a supportive sponsor, there will be no budget or end-users for the application. Here are the three most frequently encountered business challenges:
π©βπΌ Lack of business involvement:
The success of a project hinges on active engagement from business stakeholders. When this participation is lacking, there’s a significant risk of developing solutions that do not align with the broader organisational goals. Receiving constructive business feedback shows a clear interest and value in what is being created and should be treasured and embraced.
Example: A bank implements an AI-powered credit scoring model without adequate input from business leaders. The model, while capable of analysing vast amounts of data to assess credit risk, may overlook crucial factors specific to the bank’s customer base and lending policies.
π No baseline to compare to:
Without a well-defined baseline, the actual impact of an AI solution may be unclear. Demonstrating the value of an AI solution involves showing improvements over the current method. If you can’t measure the improvement quantitatively, it becomes challenging to show tangible value and support the implementation of the new approach. A baseline allows you to quantify ROI easily, clarifying business investments in ML projects.
Example: An AI-based medical image diagnosis requires a baseline accuracy rate of a radiologist’s diagnoses to compare against the existing process. Without this baseline, it’s challenging to accurately assess the effectiveness of the model and justify its integration into clinical practice.
π Short-term thinking:
While quick wins are enticing, overemphasising short-term gains without considering long-term scalability and adaptability can lead to the accumulation of technical debt. Striking a balance between immediate results and a strategic, long-term perspective is important for sustaining the success of ML projects.
Example: A machine learning model for customer churn prediction can result in technical debt due to hard-coding design components and minimal documentation. This can result in significant refactoring and cost especially if key people leave the team.
Challenge 2: Process
Process challenges are often the most difficult to address because they require altering established human behaviors. However, no AI initiative can succeed without solid processes for implementation, maintenance, and oversight. Here are the three main process challenges that frequently hinder AI projects.
π Data & Model Governance:
Effective governance is critical in managing data and AI models. Inadequate governance can lead to biased outcomes, raise ethical issues, and obscure decision-making processes in models. Furthermore, neglecting governance until the late stages of the development cycle often obstructs project completion.
Example: A financial institution’s credit scoring model reinforcing biases in historical data could result in discriminatory lending practices.
π€ Lack of cross-functional teams:
The collaboration between domain experts, engineering teams, and data scientists is crucial for successful ML projects to be developed, deployed, and operationalised. When cross-functional teams are absent, there is a risk of disjointed efforts, hindering effective problem-solving, integration, and adaptation of machine learning solutions within the organisationβs operations.
Example: A business-driven project without data science input may have a flawed scope, while a data science-driven project lacking engineering support struggles with deployment, delaying project completion.
π Sustaining model performance:
ML models must continuously adapt to changing data, trends, and business needs to maintain effectiveness. Failure to keep model performance at required levels can lead to degraded model accuracy, resulting in poor decision-making, increasing costs and customer churn through degraded service delivery.
Example: An outdated recommendation system in e-commerce can result in decreased sales and lost market share as it fails to reflect current consumer preferences.
Challenge 3: Technology and Data
AI systems are complex, combining the intricacies of standard software systems with the added challenges of large-scale data and models. This makes operationalizing AI systems a particularly tough challenge. While numerous technology and data hurdles exist, here we highlight the top three that are frequently underestimated.
π§ Data Quality and Management:
Effective data governance is essential for AI/ML projects. Challenges arise when data are poorly formatted, scattered across various sources, or of low quality. These issues can significantly compromise the accuracy of machine learning models.
Example: In a healthcare analytics project predicting patient outcomes with machine learning, the team faced data quality challenges. The dataset, sourced from multiple places, had diverse formats, incomplete entries, and inconsistent quality. These issues hindered accurate predictive modelling, risking misleading conclusions due to data noise. π₯
π Technology Stack Inefficiencies:
Each AI/ML project often begins from scratch, leading to a lack of standardisation, duplication of efforts, and a drift in technology stacks. This makes it difficult to maintain diverging models and pipelines and presents challenges in onboarding new team members efficiently.
Example: Managing different versions of machine learning frameworks like PyTorch and TensorFlow, sometimes across on-premises and various cloud environments, can become a logistical nightmare. This fragmentation can significantly delay project timelines and increase the complexity of deployments. βοΈ
π§© Maintaining Model and Pipeline Integrity:
Keeping machine learning models and their associated data pipelines consistent and up-to-date is a challenge due to the rapidly evolving nature of technology and business requirements. This can lead to discrepancies in model performance and difficulties in ensuring that the entire AI system remains coherent and aligned with current objectives.
Example: An organisation might struggle to update its sales forecasting models in line with new market conditions if its data pipelines are not designed to adapt to changes efficiently. This can result in outdated predictions and missed opportunities. π
What then?
Each business is unique. However, we’ve gathered some insights from working with 10+ data science teams.
1οΈβ£ Share knowledge and REUSE: Sharing and reusing ML assets, as exemplified by a startup’s 30% reduction in development time, directly addresses business challenges by optimising resources and accelerating time-to-market.
On the tech side, it enhances development efficiency and ensures consistency, while in processes, it streamlines workflows and facilitates knowledge transfer. This practice offers tangible solutions to various challenges encountered in AI and ML projects.
2οΈβ£ Form a great TEAM: Creating a dynamic team with a diverse skill set, as seen in a recent project transitioning seamlessly from data preprocessing to model deployment, addresses business challenges by providing comprehensive expertise.
This approach also tackles tech challenges through adaptability and fosters a culture of continuous improvement to address process challenges efficiently. π³
3οΈβ£ Involve Business: Involving stakeholders throughout development aligns solutions with user expectations, addressing business challenges by staying user-centric and market-relevant. It tackles tech challenges through adaptability and ensures effective communication, mitigating process challenges through iterative improvements. π
4οΈβ£ Iterative DEVELOPMENT: Starting with heuristics and an MVP addresses business challenges by enabling quick market entry and iterative improvements for customer satisfaction. It tackles tech challenges through optimised model accuracy and resource-efficient development while addressing process challenges through a user-centric and agile approach. π
5οΈβ£ Focus on VALUE: Prioritising features for a better end-user experience addresses business challenges by boosting monetisation and customer satisfaction. It tackles tech challenges through efficient resource allocation and continuous improvement while addressing process challenges by fostering user-centric development and effective decision-making. π‘
Conclusion
Finally, for every data science, machine learning, and AI use cases, always ask…
“Does it solve a practical problem? Does it create tangible value?”
Remember, successful AI/ML solutions create substantial worth.
. . .
Thanks for reading. If you want to know more about cloud-native tech and machine learning deployments, email us at poke@melio.ai.